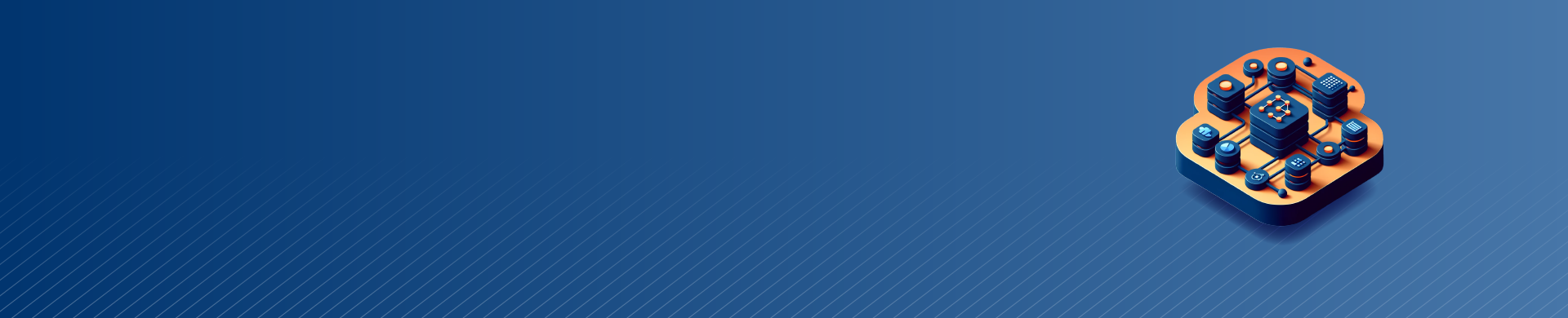
Data Management Framework
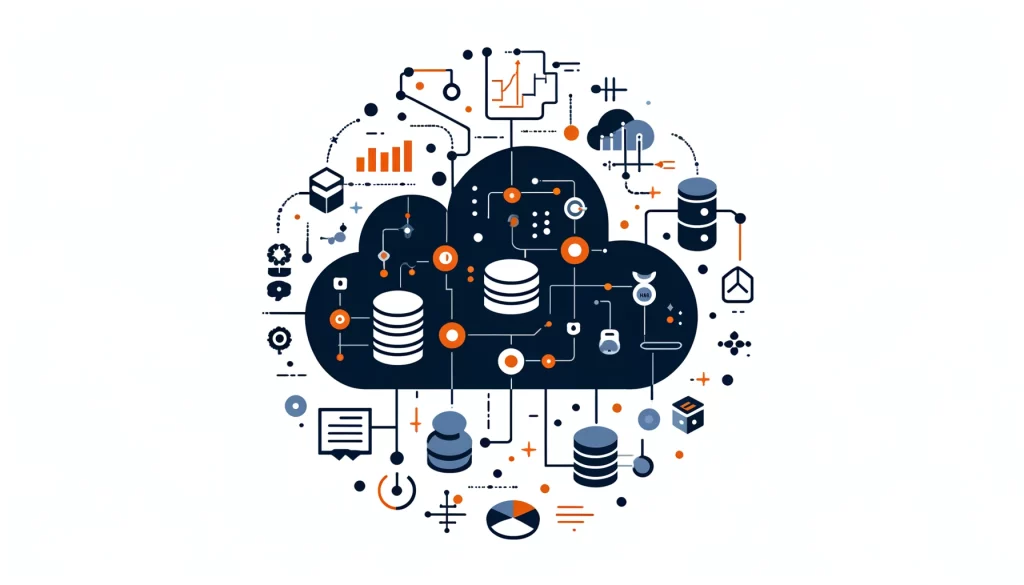
A data management framework provides a structured approach to handling data effectively. It encompasses the people, processes, and rules needed to successfully manage large amounts of information.
A data management framework is like a plan for how a company controls, protects, and uses its data.
It explains who does what, sets rules and guidelines, and lists the tools and technology needed to manage data.
The Importance of Data Management Framework
Every company has unique business drivers that determine its success. These drivers shape how closely an organization must regulate and manage its data.
A healthcare provider may focus on keeping patient information private. They also ensure that medical records are easily accessible to healthcare professionals.
These requirements form the foundation of the provider’s data management strategy and framework.
A well-designed data management framework ensures that data is trusted, well-documented, and easily discoverable within the organization. It also maintains data security, compliance with regulations, and protection from unauthorized disclosure.
The benefits of implementing a data management framework extend beyond just data governance.
By establishing a clear structure for managing data, organizations can improve data quality, reduce duplication and inconsistencies, and enable better decision-making.
A framework also helps break down data silos, promoting collaboration and data sharing across departments.
Top-Down vs. Bottom-Up Approaches to Data Management Framework
Traditionally, data management frameworks have been developed using either a top-down or bottom-up approach. The top-down method of data management involves a small group of experts who follow clear procedures and best practices.
This approach prioritizes data modeling and governance before making data widely available for analytics.
While effective, the top-down approach faces scalability issues. Small groups of data creators are struggling to meet the needs of data users in the current era of cloud computing. This is because the data creators are finding it difficult to keep up with the demands of the data users.
Cloud computing has grown quickly, making it hard for small groups to manage and share data with users. As a result, there is a gap between the data creation process and the data utilization process.
This can lead to bottlenecks and delays in accessing critical data, hindering an organization’s ability to make timely decisions.
On the other hand, the bottom-up approach offers greater flexibility. It starts with unstructured data and builds structures on top of it after ingestion.
This method is popular thanks to big data. The method is easier to expand, but it can be difficult to control and maintain data quality.
The bottom-up approach makes it easier for business users to access and work with data. However, it can also create a disorganized data environment.
Without proper governance and control, organizations may struggle with data inconsistencies, duplication, and security risks.
Striking a Balance: A Modern Approach to Data Management Framework
A new way of managing data is needed to solve the issues with old methods. This new method should strike a balance between allowing access and maintaining control.
This means taking control early in the process. Users and subject matter experts still have ownership and curation roles over the data.
An example would be a global e-commerce company that put in place a data management framework. This framework helped business users access and analyze data easily. It also allowed for centralized control over data quality and governance.
This approach enabled the company to make decisions quickly while ensuring data integrity and compliance.
A balanced data management framework should include the following key components:
- Data Governance: Establishing clear policies, standards, and processes for managing data throughout its lifecycle.
- Data Architecture: Designing and implementing the technical infrastructure to support data storage and processing.
- Data Security and Privacy: Ensuring the privacy, integrity, and availability of data assets and complying with relevant regulations.
- Data Quality: Implementing processes and tools to ensure data accuracy, completeness, and consistency.
- Data Integration: Enabling the seamless flow of data across systems and applications.
- Data Accessibility: Providing easy and secure access to data for authorized users.
Organizations can build a robust data management framework by implementing these components. These components will meet the needs of both data producers and consumers. This framework will be strong and adaptable.
Best Practices for Effective Data Management Frameworks
To create a successful data management framework, organizations should follow these best practices:
Ensure Convenient Access to Data
Providing easy access to a large amount of information enables data scientists and business analysts to make better predictions.
A comprehensive data management framework should offer native data access capabilities that simplify interaction with data from various sources, formats, and structures.
Equip the Framework with Advanced Analytics
Integrating advanced statistical analyzing capabilities into the data management process can greatly enhance data quality and insights.
For instance, frequency analyzing can identify outliers and missing values that may distort metrics like mean, average, and median.
Incorporating machine learning and artificial intelligence techniques can further augment data management frameworks.
These technologies can automate data quality checks, detect anomalies, and provide predictive insights.
Reduce Irrelevant Data
Up to 40% of strategic processes fail because of insufficient information. By implementing data cleansing directly into the data integration flow using a data quality platform, organizations can remove erroneous data and enhance data relevance.
This improves performance and supports more accurate analytics.
Data profiling techniques can help identify irrelevant or redundant data, allowing organizations to streamline their data assets. This not only saves storage costs but also improves data processing efficiency and analytics accuracy.
Foster a Proper Culture
Implementing a data management framework is not just a technical exercise; it also requires a cultural shift. Organizations must foster a culture that values data as a strategic asset and encourages data-informed decision-making.
This involves providing training and support to employees, promoting data literacy, and recognizing and rewarding respective initiatives. Leaders should lead by example, demonstrating the value of data in their decision-making processes.
Continuously Monitor and Improve
A data management framework is not a one-time implementation; it requires ongoing monitoring and improvement. Organizations should establish metrics and key performance indicators (KPIs) to measure the effectiveness of their data management processes.
Regular audits and assessments can help find ways to improve and make sure the framework stays in line with business goals. As data volumes and complexity grow, organizations must continually adapt and optimize their data management practices.
Real-World Examples of a Successful Data Management Framework
Many organizations have successfully implemented data management frameworks to drive business value. One notable example is a global financial institution that developed a framework to manage its complex data landscape.
The institution made data better, lowered risks, and helped with decision-making. They did this by giving clear roles, explaining data, and creating strong data processes. This was done by establishing clear roles and responsibilities within the organization.
They also standardized data definitions to ensure consistency and accuracy. Additionally, robust data governance processes were put in place to manage and protect the data effectively.
A healthcare provider used a system to collect information from different sources and make a full patient profile. This framework allowed clinicians to access comprehensive patient information, leading to improved care coordination and better patient outcomes.
A grocery store company used a system to learn more about what customers like and how they behave.
The company collected data from loyalty programs, point-of-sale systems, and social media. They used this data to personalize marketing campaigns. They also used it to improve supply chain operations.
This led to increased customer satisfaction, higher sales, and reduced waste.
Conclusion
In today’s competitive business environment, organizations that harness the power of data through effective management frameworks gain a significant advantage.
Companies can benefit greatly by implementing best practices and finding a balance between access and control. This can help them unlock valuable insights, improve decision-making, and drive innovation.
As data volumes continue to grow, the importance of robust data management frameworks will only increase. Organizations can capitalize on future opportunities by investing in the right tools, processes, and people.
However, implementing a data management framework is not a one-size-fits-all solution. Each organization must tailor its approach to its unique business needs, regulatory requirements, and data landscape.
This requires a deep understanding of the organization’s data assets, business goals, and stakeholder needs.
In the future, data management will be influenced by new technologies like artificial intelligence, blockchain, and edge computing.
New technologies will make collecting, organizing, and analyzing data easier. This will highlight the importance of having good data management skills.
Organizations that focus on data management frameworks will be in a better position to maximize their data assets. This will help them navigate the complex data landscape effectively. By prioritizing data management, organizations can unlock the full potential of their data assets.
Companies can benefit from treating data as a strategic asset. This can drive innovation and improve customer experiences. It can also help them gain a competitive edge in the marketplace. Investing in effective management practices is key to achieving these benefits.