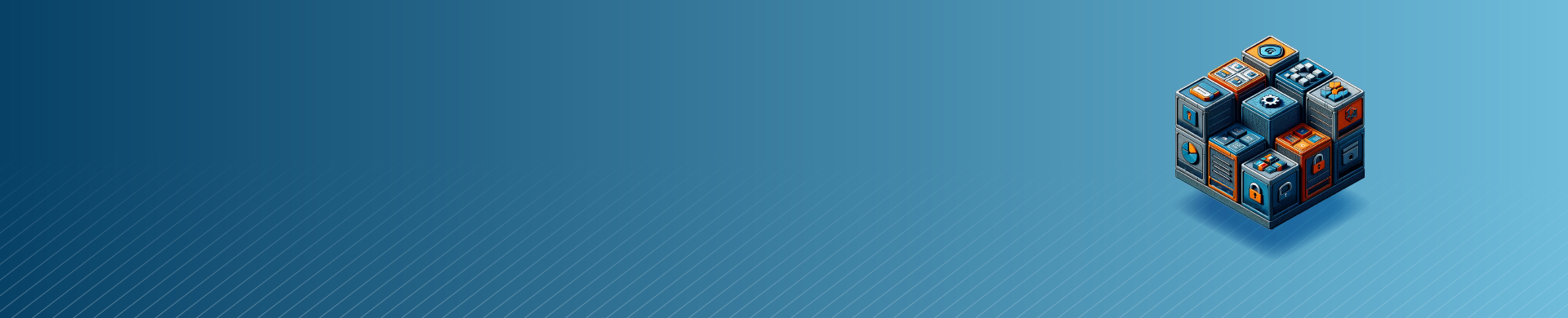
Data Classification Types
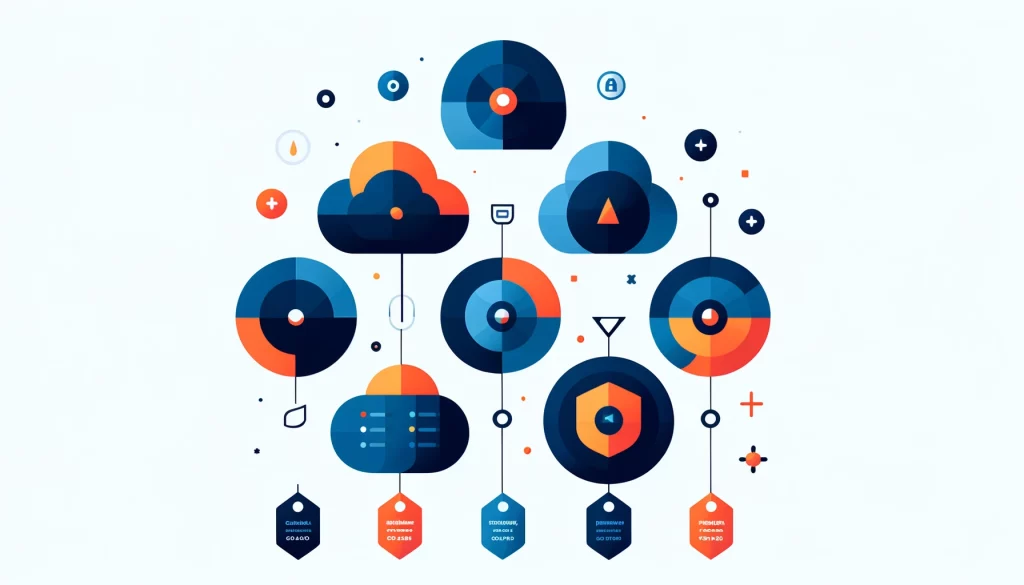
Data classification is a critical process for organizing structured and unstructured data into categories and it has different types. The goal is to use data more securely and efficiently.
Data classification makes it easier for organizations to find and access their data. It also enables better risk management, regulatory compliance, and legal discovery.
Data classification processes involve labeling personal information and sensitive data. These labels help effectively search and track data. Data classification also removes duplicate data, lowers storage and backup costs, and helps reduce cybersecurity risks.
Data Classification Criteria
Content-Based Data ClassificationOne common data classification type is content-based classification. This method assigns labels based on the contents of data elements. It reviews information stored in databases, documents, and other sources. It then applies tags that define the data type and sensitivity level.
Content-based classification directly examines the data itself to determine how it should be categorized. By looking at the actual contents, it aims to accurately classify data according to predefined criteria. This helps ensure we properly identify and handle sensitive information.
Context-Based Data ClassificationAnother key data classification type is context-based classification. Rather than looking at content, this method uses contextual details like metadata to classify data. For instance, it might automatically tag all files from a certain application or user as financial data.
Context-based classification can also use predefined rules to generate labels. These rules define the data type and sensitivity based on associated information, without scanning content.
This allows large volumes of data to be classified based on their source, creator, or other characteristics.
User-Based Data ClassificationA third core data classification type is user-based classification. In this approach, a knowledgeable user decides which label to apply to a data element. This user could be a dedicated classification authority or the original data creator.
User-based classification taps into a person’s expertise about the data. A user familiar with the information can assess its business value and sensitivity to determine the right categorization. They understand the full context and can make nuanced classification decisions.
However, user-based classification can face scaling challenges in organizations with large data volumes. Manually tagging each piece of data may not be feasible.
Users often combine user-driven classification with automated methods in an overall data classification strategy.
Business Data Sensitivity Levels
When classifying data, businesses commonly use several data sensitivity levels:
Restricted data is highly sensitive information with strict access controls. This might include intellectual property, personal information, trade secrets, health data, and payment card information. Unauthorized disclosure of restricted data can have serious financial or legal consequences.
Confidential data has broader access within an organization but is still internal. Legal restrictions on handling are often present. Examples include pricing, contracts, and marketing plans. Disclosing confidential data can negatively impact business operations and brand reputation.
Internal data is available company-wide but still requires some protection. Items like employee directories, memos, and handbooks fall under this category.
Public data does not need security controls and one can freely share it.
Government Data Sensitivity Levels
Government organizations frequently use another set of data sensitivity levels:
Top Secret information has the highest protection and access restrictions. Its disclosure could threaten national security.
Secret data also requires strong safeguards, as it could seriously damage national security if released.
Confidential is the lowest level of classified government data. It still requires solid protection, but less than Top Secret or Secret data.
Sensitive but unclassified (SBU) data is information that is not classified but still needs protection. This protection is necessary to prevent the violation of citizens’ privacy rights.
Unclassified data is to conside non-sensitive.
Establishing a Data Classification Policy
To put data classification into practice, organizations need to define a clear policy. This policy explains how employees should manage different types of data to ensure data security and meet management goals.
A good policy helps users easily understand how important information is and what rules to follow.
Paper-based Data Classification PolicyPaper-based classification policies rely on well-documented guidelines and employee training to ensure proper data categorization and handling.
These policies explain the various levels of data classification (restricted, confidential, internal, public). They also provide clear instructions on how to label, store, and share each type of data.
To implement a paper-based policy effectively, organizations must invest in comprehensive employee education programs. This means starting training sessions to explain the classification guidelines. It also involves having regular refresher courses to review important concepts. Additionally, these courses address any policy updates or changes.
Employees need practical examples and hands-on exercises to understand how to apply classification rules to their job roles.
However, the success of a paper-based policy heavily depends on employee compliance and consistency. Without automated enforcement mechanisms, it’s up to individual users to manually classify and label data correctly. This can be time-consuming and prone to human error, particularly in organizations with large volumes of data or high employee turnover rates.
Automated Data Classification PolicyAutomated data classification policies leverage software algorithms and machine learning techniques to analyze and categorize data based on predefined rules and patterns. These tools can scan data from different sources like databases, file servers, and cloud storage. They can then automatically assign labels based on the content and context of the information.
One key benefit of automated classification is its ability to enforce policies consistently and at scale. After defining the classification rules, the software will apply them consistently throughout the organization. This helps minimize errors and ensures that all data is correctly categorized and protected. This is particularly valuable for organizations with large and complex data environments, where manual classification would be impractical or impossible.
However, automated classification is not without its challenges. These tools use set rules and algorithms. However, they may struggle to grasp the full context and subtleties of specific data types. This can cause mistakes, where data is either wrongly labeled as sensitive or not identified and protected as sensitive.
User-Driven Data Classification PolicyEmployees can make decisions about the sensitivity and value of the data they work with. Implement user-driven classification policies to achieve this. These policies empower employees to make informed decisions.
These policies provide users with classification levels and guidelines. Users must use their judgment and expertise to determine how to categorize each piece of data.
User-driven classification can also help foster a culture of data security and privacy awareness within the organization. These policies help employees understand how to protect data and encourage them to take responsibility for safeguarding sensitive information. They prompt employees to actively think about and categorize the data they work with. This can increase awareness of best practices for data protection.
When users have to organize things themselves, it can be time-consuming and make it difficult for employees to work efficiently. This is especially true when the organizing process does not match well with the tools and methods they are already using. This is especially true if the organizing process doesn’t work well with the tools and ways they already use. Organizations must strike a balance between involving users in the classification process and minimizing the burden on their productivity.
Integrating Data Classification with Other Security Measures
Data classification is a critical component of an overall data security strategy, but it should not exist in isolation. Integrating classification with other security measures can create a more comprehensive and effective approach to protecting sensitive information.
For instance, data loss prevention (DLP) solutions can leverage classification labels to enforce policies around data usage and transmission. If a user attempts to send a file classified as confidential outside the organization, the DLP system can automatically block the transfer and notify security teams.
You can also tie access controls to classification levels. The system grants users different permissions based on the sensitivity of the data they need to work with. This measure makes sure that only authorized people can access or change sensitive information. It reduces the risk of accidental or intentional data breaches.
Encryption is another security measure that can be applied based on classification. To add an extra layer of protection, highly sensitive data can be automatically encrypted both when stored and when being transmitted. By integrating classification with encryption, organizations can ensure their most critical assets receive the strongest level of security.
Conclusion
In summary, data classification types include content-based, context-based, and user-based approaches. Organizations use sensitivity levels like restricted, confidential, internal, and public. Implementing a data classification policy through manual, automated, or user-driven methods enables more secure and streamlined data management.
However, effective data classification is not a set-and-forget endeavor. Regular reviews and audits are crucial to ensure the classification system remains accurate and relevant over time. As data and business needs evolve, classification policies must adapt accordingly. Ongoing employee training is also essential to maintain a strong data security culture and ensure consistent policy adherence.
Looking ahead, the future of data classification is likely to be shaped by advancing technologies like machine learning and artificial intelligence, as well as the growing adoption of cloud computing and remote work models.
These changes will create opportunities and challenges for organizations trying to keep their data safe in a more complex digital world.
Strong data classification strategies are essential for all organizations, regardless of size or industry. Data plays a key role in driving business growth and decision-making. Therefore, investing in effective data classification strategies is crucial.
Companies can get the most out of their data and keep stakeholders’ information safe by using best practices for data classification. To achieve these goals, it is important to understand the different types of data classification. Understanding the various types of data classification is key to achieving these goals.